Official project site of Semantic-Aware Scene Recognition (Elsevier Pattern Recognition 2020).
Abstract
Scene recognition is currently one of the top-challenging research fields in computer vision. This may be due to the ambiguity between classes: images of several scene classes may share similar objects, which causes confusion among them. The problem is aggravated when images of a particular scene class are notably different. Convolutional Neural Networks (CNNs) have significantly boosted performance in scene recognition, albeit it is still far below from other recognition tasks (e.g., object or image recognition).
In this paper, we describe a novel approach for scene recognition based on an end-to-end multi-modal CNN that combines image and context information by means of an attention module. Context information, in the shape of a semantic segmentation, is used to gate features extracted from the RGB image by leverag- ing on information encoded in the semantic representation: the set of scene objects and stuff, and their relative locations. This gating process reinforces the learning of indicative scene content and enhances scene disambiguation by refocusing the receptive fields of the CNN towards them.
Experimental results on three publicly available datasets show that the proposed approach outperforms every other state-of- the-art method while significantly reducing the number of network parameters.
Proposed Method
The architecture is composed of a Semantic Branch, a RGB Branch and an Attention Module. The semantic branch aims to extract meaningful features from a semantic segmentation score map. This Branch aims to convey an attention map only based on meaningful and representative scene objects and their relationships. The RGB Branch extracts features from the color image. These features are then gated by the semantic-based attention map in the Attention Module. Through this process, the network is automatically refocused towards the meaningful objects learned as relevant for recognition by the Semantic Branch. Better viewed in color.
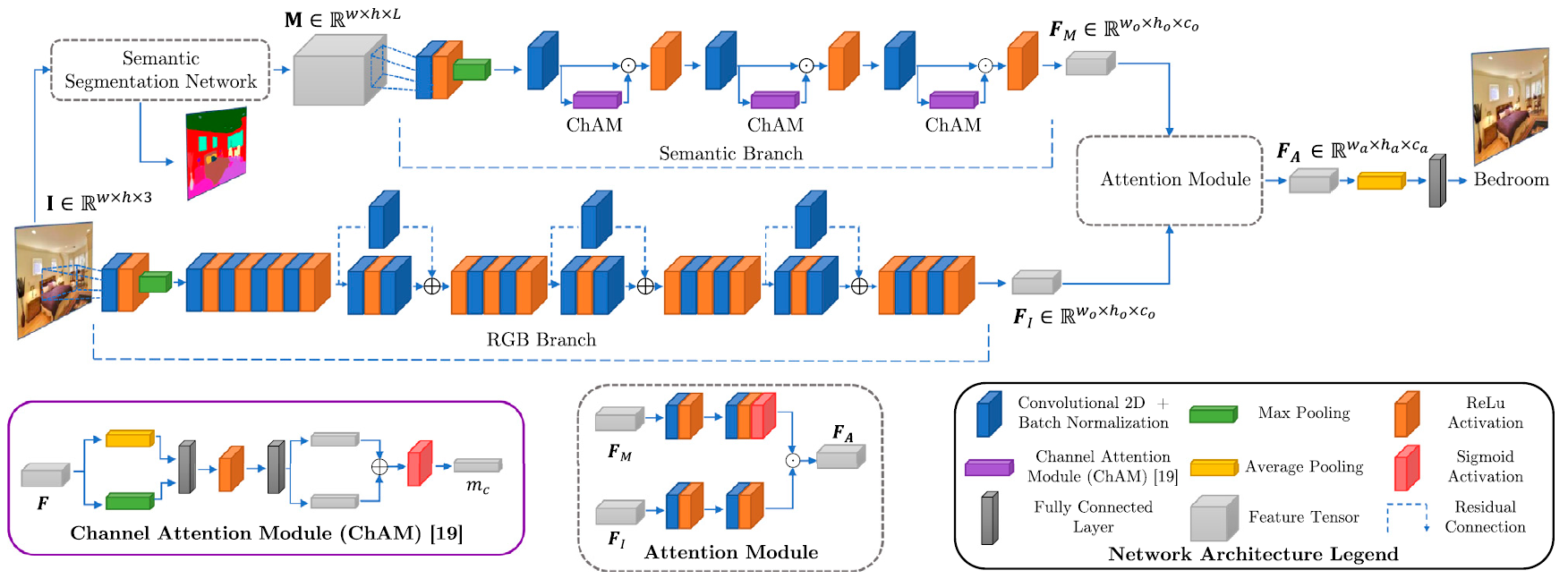
Results
Results on Places365 dataset. The proposed method (Ours) obtains the best results while maintaining relatively low complexity. Its performance improves those of the deepest network, DenseNet-161, by a 0.73% in terms of Top@1 accuracy and it surpasses the highest-capacity network, VGG-19, by a 2.29% reducing the number of parameters a 67.13%.
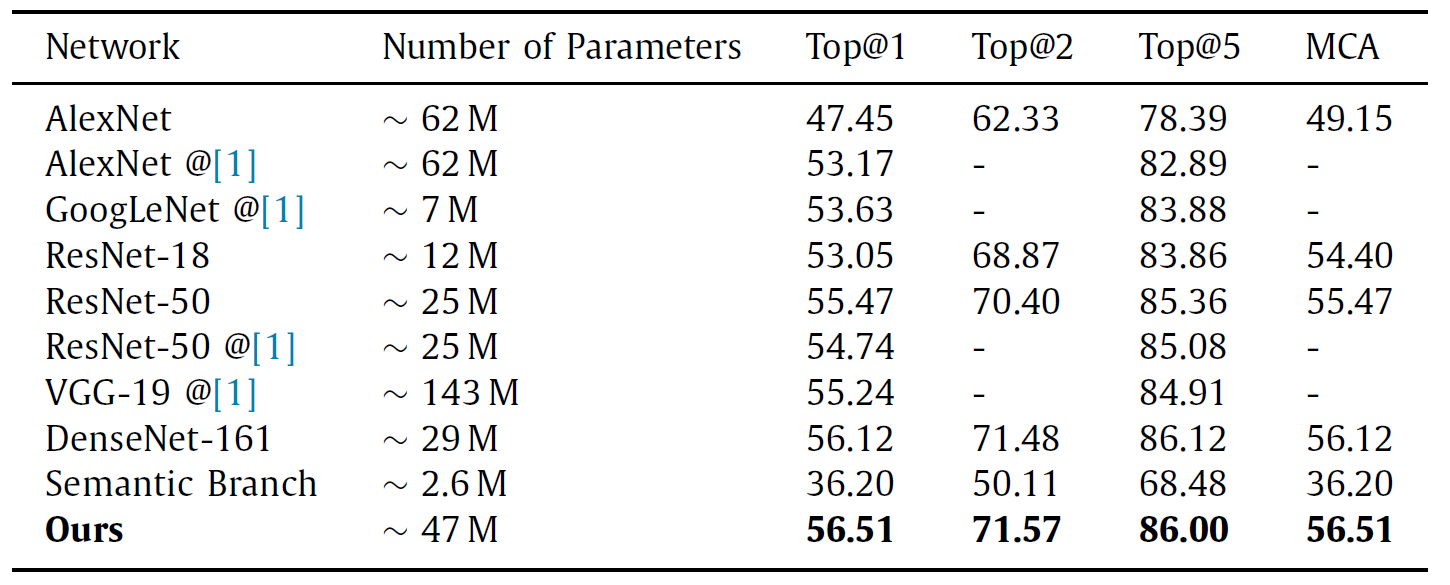
Related Work
López-Cifuentes, A., Escudero-Viñolo, M., Bescós, J., & San Miguel, J. C. (2022). Using a DCT-driven Loss in Attention-based Knowledge-Distillation for Scene Recognition.
Comment: Using DCT transform to enhance Attention-based Knowledge-Distillation for Scene Recognition.
López-Cifuentes, A., Escudero-Viñolo, M., Gajic, A., & Bescós, J. (2021). Visualizing the Effect of Semantic Classes in the Attribution of Scene Recognition Models. In Proceedings of ICPR International Workshops and Challenges.
Comment: Visualizing the attribution of scene recognition models by perturbing the in input images with semantic segmentation.
Citation
If you find this work useful, please consider citing:
López-Cifuentes, A., Escudero-Viñolo, M., Bescós, J., & García-Martín, Á. (2020). Semantic-aware scene recognition. Pattern Recognition, 102, 107256.
@article{lopez2020semantic, title={Semantic-aware scene recognition}, author={L{\'o}pez-Cifuentes, Alejandro and Escudero-Vi{\~n}olo, Marcos and Besc{\'o}s, Jes{\'u}s and Garc{\'\i}a-Mart{\'\i}n, {\'A}lvaro}, journal={Pattern Recognition}, volume={102}, pages={107256}, year={2020}, publisher={Elsevier} }
Acknowledgement: This study has been partially supported by the Spanish Government through its TEC2017-88169-R MobiNetVideo project.
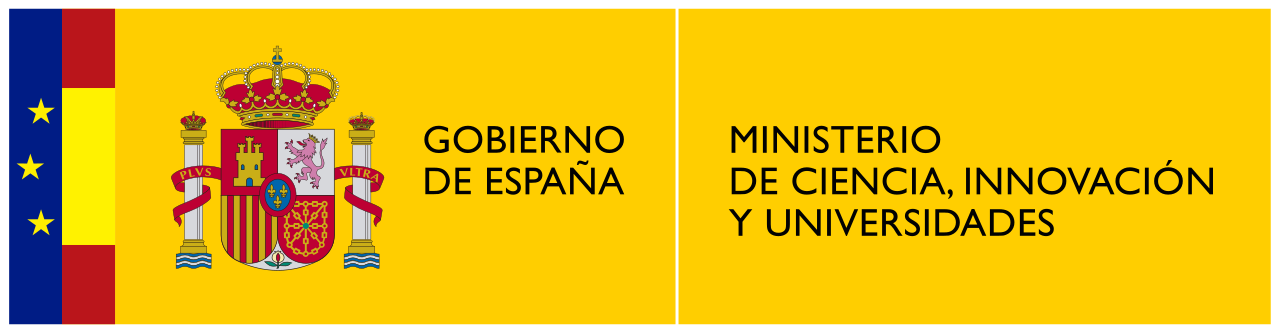